Overwatch League (OWL) Data Analysis
Context
This final group project in Business Analytics allowed me and my team to apply our analytical knowledge and skills to a real‐world problem that was of interest to at least one person in our group. The objective was not to solve the problem or to come up with an analysis that was going to change the world—we simply did not have the time or resources within the scope of this project to do anything of much practical value. However, we could use this opportunity to do a superficial analysis of a real‐world problem and, in the process, learn about how we might go about such an analysis as a full‐time/full‐resources undertaking.
The Task
Our task was ultimately to conduct a Root Cause Analysis using a continuous response variable and multiple potential explanatory variables. We needed to source our own real-world data and could use any of the analytical/statistical tools and techniques we'd learned to use throughout the course. I pitched my idea of using esports (Overwatch League) performance data to determine the "root cause" of what makes a "good" (profitable) player, and both my team and the prof really liked this idea (despite the ambitious scope, given our time/resources).
The Problem
The fundamental problem analyzed in this project was what player performance metrics could be relied on to predict the OWL players who are most likely to increase their team’s profitability through prize money earnings. For decision-makers at the esports organizations that own and operate OWL teams, this information could be extremely valuable. Multilinear regression was used in SAS Enterprise Guide, with the support of KNIME regression tree to identify and include critical explanatory variables in the model. We collected player prize money earnings data from esportsearnings.com and in-game player performance data from the official OWL Stats Lab*.
The Result
Based on our analysis, we concluded predicting prize money earnings based on publicly available player performance data presents significant challenges. Our best model suggested that a player's "Damage Taken," "Ultimates Earned (Fractional)," and "Ultimates Used" would be most accurate in determining the highest earning players. However, the predictive models can only be used as reference for making decisions, as the R-squared for both methods only explained ~27% of the total prize money earnings we analyzed. More specifically, there are significant challenges in missing values, inconsistent data collection, inherent complexity in the domain (roles, metas), and changes in the game's fundamental structure (Overwatch 1 to Overwatch 2, 6v6 to 5v5). Thus, we concluded that experienced talent scouts, internal/private earnings data, or more complex and resource-intensive predictive models would be necessary to establish more reliable explanatory variables.
*note: the Stats Lab has been deprecated since the dissolution of the OWL (now replaced by "Overwatch Esports")
Rows of Performance Data Compiled
Players' Earnings Analyzed
Final Mark
Tools Used
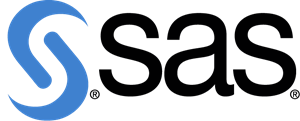
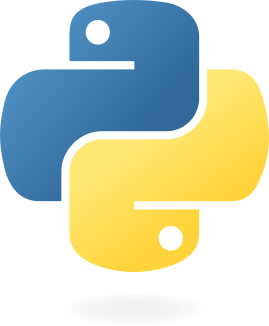